Safeguarding AI
The safeAI team of IABG is developing solutions for the evaluation, testing, and conformity assessment of Artificial Intelligence (AI) systems.
We combine state-of-the-art AI research, standardization, and regulation with the vast experience of IABG in testing, analyses, and certification processes. By actively participating in AI standardization committees on national, European, and international level, we support the development of new standards and guidelines to increase the benefits of AI systems. To support our developments related to uncertainty quantification, we have initiated the DIN SPEC 92005 "Uncertainty quantification in machine learning".
Contact us to learn more about our activities and ways for cooperation. We look forward to working together with you to create beneficial and trustworthy AI technologies.
Dimensions of AI Safety Assessment

Dataset
Dataset analysis involves examining the underlying data of an AI model to assess its balance and representativeness for the given application context. Additionally, weaknesses or underrepresented data points are identified to ensure optimal model performance.

Performance
Performance evaluation is the process of determining the effectiveness of a model in carrying out its designated tasks. Quantifying model performance using application-specific metrics is essential in guiding the decision to deploy the AI model and identifying the necessity of additional safety measures.

Uncertainty
Uncertainty is an inherent part of any AI application but not all models estimate it. Correctly quantified uncertainties can be used to assess if predictions should be trusted and thus lead to better decision-making and increased safety.

Robustness
Robustness evaluation investigates the AI model's resilience towards various types of input perturbations. Safeguarding AI implies that a model maintains its level of performance under varying conditions in real-world scenarios.

Explainability
The safe application of an AI model and its resulting decisions require the traceability and interpretability of the outputs. By considering the explainability of the model, predictions and decisions become transparent and traceable.

Compliance
Compliance refers to the act of adhering to requirements and rule sets for building and use of safe and trustworthy AI systems. AI standards specify requirements that relate to AI evaluations as the basis of the compliance process. The rulesets are specified by regulations such as the European AI Act as well as the AI standards.
safeAI in Action
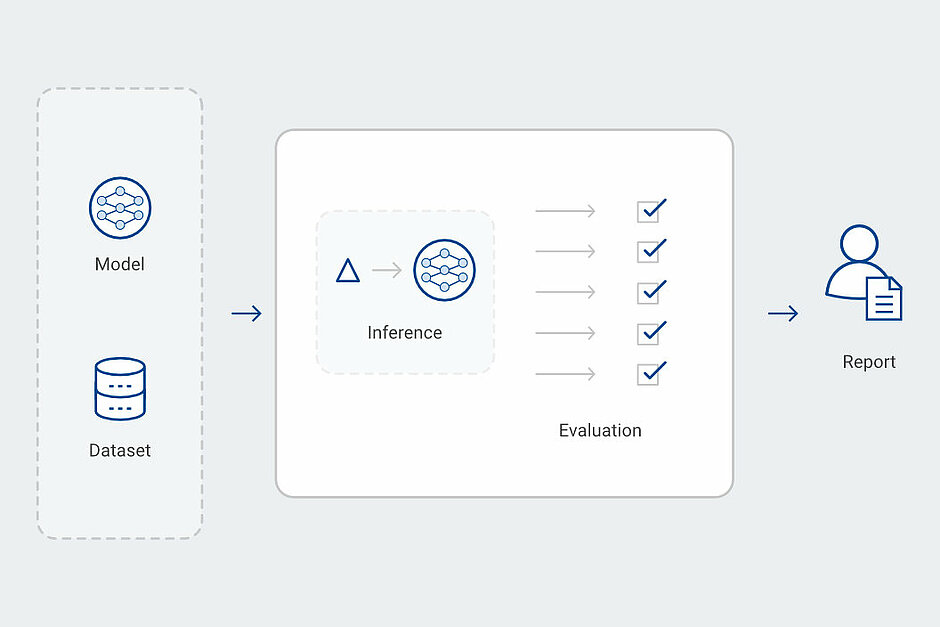
AI Evaluation Workflow
Assessing a given AI model and its underlying dataset with respect to safety and reliability requires an infrastructure for multi-level evaluation.
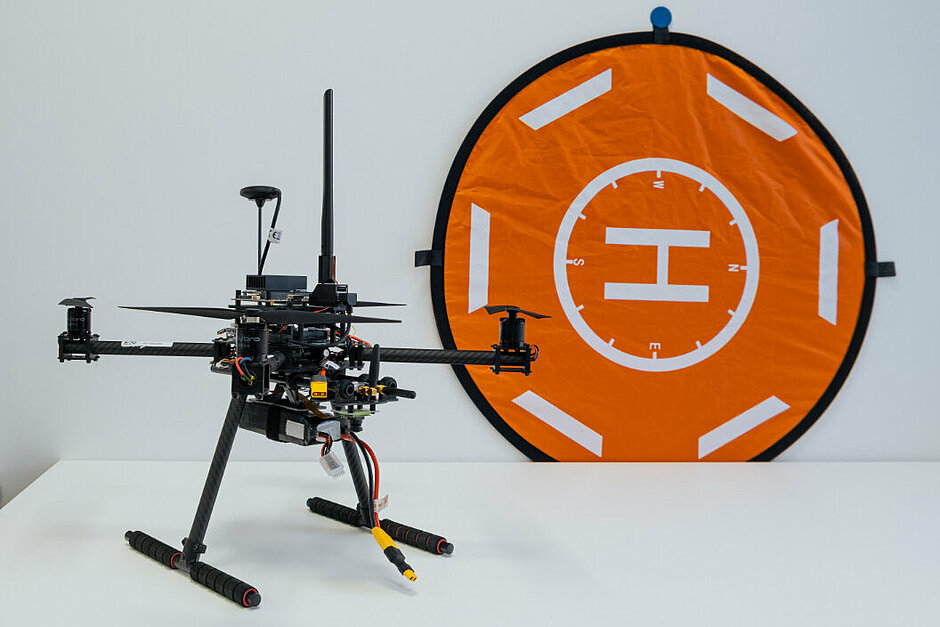
Software/Hardware Integration
Integration of AI-based software components into a cyber physical system needs extensive testing in virtual and real settings before it can be deployed safely.
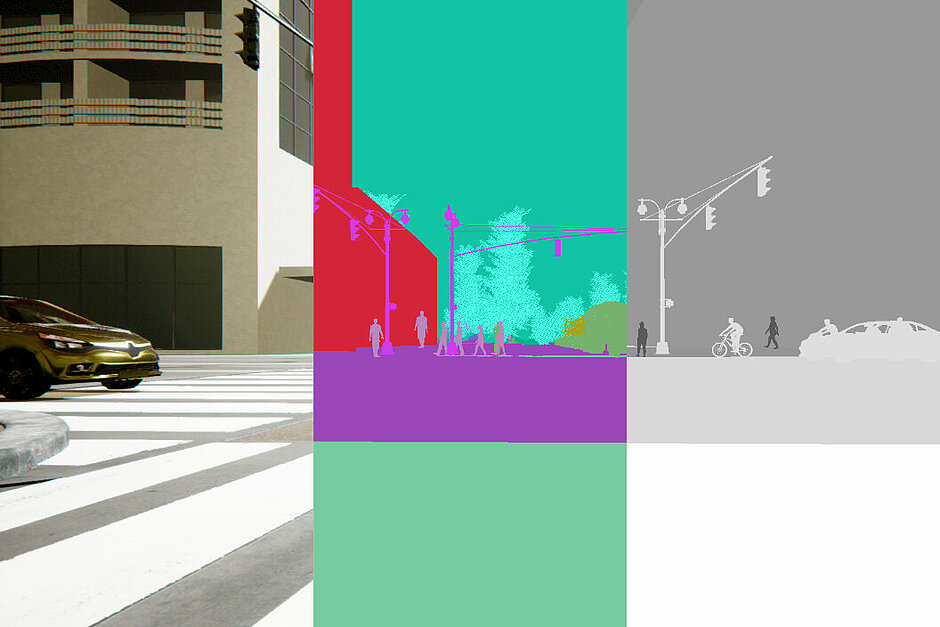
Synthetic Data Generation
Photo-realistic simulation environments allow data collection, labeling, and augmentation for training and evaluation of AI.